What Is Science Data Analysis: Examples, Types, and Applications
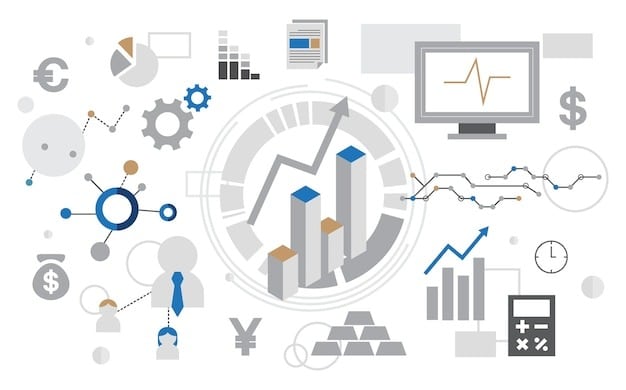
What Is Science Data Analysis: Examples, Types, and Applications
Discover the power of science data analysis ⚡ Dive into examples, types, and real-world applications that transform complex data into actionable insights.
In today’s data-driven world, companies and researchers alike rely on data science and data analysis to make informed decisions, predict trends, and gain insights into complex problems. As a leader in customer interaction, Rapid Phone Center understands the critical importance of effective data use in business and research. In this comprehensive guide, we’ll explore the key aspects of data science analysis, types of data analysis, the distinction between data science and data analysis, and specific approaches for applying data science in social science research.
Introduction to Data Science v Data Analysis
The terms data science and data analysis are often used interchangeably, but they encompass different aspects of data-driven decision-making. Data science focuses on uncovering patterns and insights through advanced computational methods, while data analysis involves examining and interpreting existing datasets to support specific objectives.
Rapid Phone Center applies these fields to understand customer behaviors, improve service offerings, and predict future trends. In this guide, we’ll address common questions such as the differences between data science vs data analysis, predictive analysis in data science, and the importance of data analysis in social science research. Mastering Sales Objections: Learn techniques to handle client objections with confidence, turning challenges into opportunities to close sales successfully.
Quantitative Analysis Data Science: Transforming Data into Insights
Understanding the difference between data science and data analysis is crucial for companies and researchers alike:
- Data Science: Encompasses the entire data lifecycle, from data extraction and cleaning to modeling and interpreting complex datasets. It uses advanced methods such as machine learning, artificial intelligence, and quantitative analysis to make predictions or discover trends.
- Data Analysis: Focuses specifically on examining data to answer specific questions, often relying on statistical or quantitative methods. This process typically follows defined steps, such as cleaning, transforming, and visualizing data, before making conclusions. Quantitative data analysis involves statistical techniques to analyze numerical data, revealing patterns and trends for informed decision-making.
Data Science vs Data Analysis Summary:
- Scope: Data science is broader and more technical, while data analysis is more focused on answering specific questions.
- Methods: Data science utilizes advanced methods (like machine learning), whereas data analysis relies on statistical approaches.
- Goal: Data science aims to uncover new insights and patterns, while data analysis interprets data to solve existing problems.
Social Science Data Analysis Software: A Comprehensive Review of the Best Tools
Rapid Phone Center leverages different types of data analysis science to optimize processes, from customer support to lead generation. Understanding each type is essential for businesses and researchers alike.
Descriptive Analysis
- Purpose: Describes historical data trends.
- Use Cases: Monitoring customer satisfaction scores, tracking call response times.
Diagnostic Analysis
- Purpose: Examines why something happened.
- Use Cases: Analyzing factors that lead to high customer churn rates or identifying trends in customer complaints.
Predictive Analysis
- Purpose: Uses historical data to predict future outcomes.
- Use Cases: Forecasting demand for customer support services, anticipating busy periods for call centers.
Prescriptive Analysis
- Purpose: Recommends actions based on predictions.
- Use Cases: Suggesting staffing levels for high-demand times or creating action plans to reduce wait times. Sales Team Management: Boost team performance with effective strategies in training, motivation, and goal-setting for outstanding sales results.
Predictive Analysis Data Science: Harnessing Data for Future Insights
The process of data analysis in science, and business involves systematic steps to ensure meaningful insights. Here’s a look at how data analysis is typically performed:
- Define Objectives: Establish clear goals, such as improving customer satisfaction or enhancing operational efficiency.
- Data Collection: Gather data from relevant sources, including call records, customer feedback, and operational data.
- Data Cleaning: Ensure data accuracy by handling missing values and eliminating inconsistencies.
- Data Analysis: Apply statistical and quantitative analysis techniques to reveal patterns.
- Interpretation and Reporting: Translate findings into actionable insights, such as improving customer engagement strategies or optimizing service processes.
At Rapid Phone Center, we specialize in data analysis social sciences, offering tailored solutions to help researchers and organizations unlock valuable insights. Our expert team utilizes advanced statistical methods and data analytics tools to analyze social phenomena, ensuring your findings are accurate and impactful. Whether you’re exploring demographics, trends, or behavior patterns, we provide the expertise you need to drive informed decisions and enhance your research outcomes. Transform your support with AI customer service solutions, providing quick responses and personalized experiences for enhanced satisfaction.
Data Analysis and Data Science in Social Science Research
Data analysis is vital not only in business but also in social science research. Through data analysis in social science research, researchers can validate theories, assess public behavior, and gain insights into societal patterns. Here’s how data science and analysis play out in social sciences:
Quantitative Analysis in Data Science and Social Sciences
- Objective: Quantitative analysis in data science allows social scientists to interpret large datasets objectively.
- Applications: Studying demographic data, analyzing social media sentiment, or exploring survey results.
Predictive Analysis in Social Science Data
- Objective: Using predictive analysis, researchers forecast social trends or behavior changes.
- Applications: Analyzing data on employment trends, public health issues, or educational outcomes.
Social Science Data Analysis Software
- Overview: Specialized software aids in managing and analyzing complex social science datasets.
- Examples: Programs like SPSS, SAS, and R, widely used for data analysis in social sciences, assist researchers in working with large, complex datasets.
Importance of Data Analysis in Social Science Research
- Data analysis enables social scientists to uncover relationships, test hypotheses, and develop evidence-based insights that contribute to policymaking and social betterment. Follow Up Call: Strengthen client relationships and boost conversions with timely, personalized follow-up calls that show commitment and care.
Challenges and Best Practices for Data Science and Data Analysis
In both business and social science, challenges in data science and data analysis may include data privacy concerns, ethical issues, and technical limitations. Rapid Phone Center ensures that data is analyzed responsibly and ethically by following industry standards. Here are some best practices:
- Data Privacy: Secure data storage and access to prevent breaches.
- Bias and Overfitting: Avoid using biased datasets and overfitting in predictive models.
- Tool Selection: Choosing the right tools (e.g., statistical software for social science data analysis) can significantly improve analysis efficiency.
Rapid Phone Center excels in data science data analysis, delivering comprehensive solutions to empower businesses and researchers. Our skilled team utilizes cutting-edge tools and techniques to transform complex datasets into actionable insights. From predictive analytics to data visualization, we help clients make informed decisions that drive growth and efficiency. Trust us to provide expert analysis that enhances your strategic initiatives and fosters data-driven success.
Maximizing the Power of Data Science and Data Analysis
Data science and data analysis offer powerful tools for understanding complex datasets, whether in a business context like Rapid Phone Center or in social science research. From predictive analysis in data science to software solutions for data analysis social science, these methodologies provide a structured approach to making informed decisions. By investing in robust data practices, companies and researchers can unlock new levels of insight, efficiency, and innovation. Efficient ecommerce fulfillment solutions streamline order processing, storage, and delivery, enhancing customer satisfaction and loyalty.
At Rapid Phone Center, we focus on data analysis in social science, offering specialized services to enhance research outcomes. Our experienced team leverages advanced analytical techniques to interpret social data, uncovering meaningful patterns and trends. We support researchers, educators, and organizations in making data-driven decisions that impact society. With our expertise, we turn complex data into actionable insights, helping you achieve your goals in social science research.
Frequently Asked Questions
Explore our Frequently Asked Questions for Science Data Analysis at Rapid Phone Center to enhance your understanding and skills! Optimize your operations with expert back office services, ensuring smooth administrative support, data management, and efficiency.
What is science data analysis?
Science data analysis involves the process of inspecting, cleansing, transforming, and modeling scientific data to discover useful information, inform conclusions, and support decision-making.
What types of data are commonly analyzed in scientific research?
Common types of data include experimental measurements, observational data, survey results, and simulated data. This can encompass numerical, categorical, time-series, and geospatial data.
What are the steps involved in data analysis?
The typical steps include data collection, data cleaning, exploratory data analysis (EDA), statistical analysis, modeling, and interpretation of results.
What tools and software are commonly used for data analysis in science?
Popular tools include programming languages such as Python and R, statistical software like SPSS and SAS, and data visualization tools like Tableau and Power BI.
You might also want to explore the offerings we provide:
Thanks, Good Luck to You 🌊