A Comprehensive Data of Analysis Report Example for Beginners
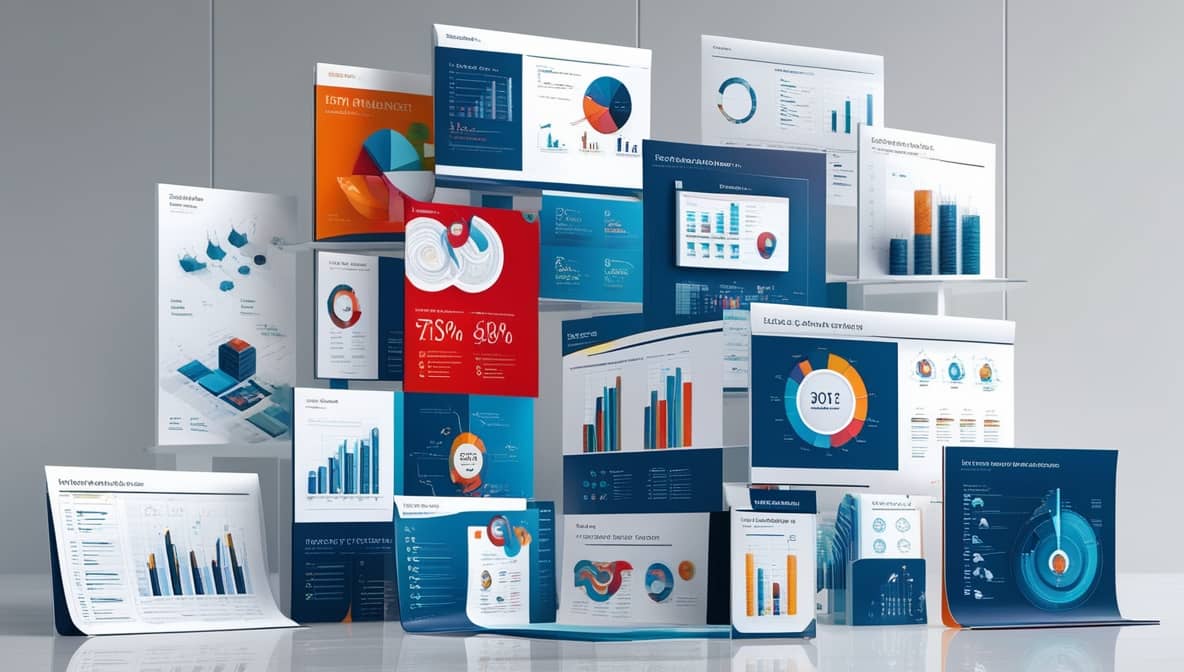
A Comprehensive Data of Analysis Report Example for Beginners
Learn how to create a professional data analysis report example with this detailed Discover key elements, structure, and tips for impactful reporting.
In the fast-paced business world, the ability to analyze data effectively and generate insightful reports is crucial for making informed decisions. Data analysis and reporting provide organizations with the tools they need to understand trends, predict future outcomes, and optimize their strategies. This guide will explore various types of data analysis reports, including examples and practical applications, with a focus on predictive analytics and big data analysis.
What is Data Analysis Reporting?
Data analysis reporting is the process of interpreting and presenting data findings in a structured format, typically within a report. The purpose is to communicate key insights to decision-makers, stakeholders, and other relevant parties. These reports are based on data collected from various sources, and they include summaries, findings, and actionable recommendations.
A well-crafted data analysis report can provide deep insights into various aspects of a business, such as sales performance, customer behavior, operational efficiency, and more. An analysis of data is the process of inspecting, cleaning, and modeling data to uncover useful information and support decision-making.
Types of Data Analysis Reports
Data analysis reports come in different formats, each serving a unique purpose. Some common types include:
- Sales Data Analysis Report: Focuses on analyzing sales trends, customer purchasing behavior, and overall sales performance.
- Big Data Analysis Report: Involves the analysis of large datasets to uncover patterns and trends that are not visible with smaller data sets.
- Predictive Analytics Report: Uses historical data and statistical algorithms to predict future outcomes, often applied in forecasting sales, customer behavior, or market trends.
- Research Report Data Analysis: A detailed examination of data collected during research, often in academic, scientific, or market research contexts.
- Data Analytics Reporting: A more general approach to analyzing various types of data to identify trends, issues, and opportunities across an organization.
Each type of report serves a distinct purpose, yet they all share the same goal of providing actionable insights to stakeholders. Exploratory Data Analysis (EDA) uncovers patterns, trends, and insights from datasets, helping guide further analysis and decision-making.
Example Data Analysis Report
Let’s break down a few examples of data analysis reports to better understand their structure and use. Software for Data Analysis helps process, visualize, and interpret data, enabling informed decision-making and efficient data-driven insights.
Example 1: Sales Data Analysis Report
A sales data analysis report example might include data such as:
- Total sales volume and revenue over a specific period.
- Breakdown of sales by product category or region.
- Analysis of customer purchasing patterns and trends.
- Recommendations for improving sales based on insights from the data.
This type of report helps businesses understand their sales performance and identifies opportunities for growth.
Example 2: Big Data Analysis Report
A big data analysis report involves the processing and interpretation of vast amounts of data to uncover hidden patterns, correlations, and insights that may not be visible through traditional data analysis methods. For instance:
- Analysis of customer data from millions of interactions across multiple touchpoints.
- Identifying trends in purchasing behavior across different demographic groups.
- Uncovering inefficiencies in business operations that could be optimized.
Big data reports are invaluable for companies that rely on massive datasets, such as e-commerce platforms or large enterprises. Types of Data Analysis include descriptive, diagnostic, predictive, and prescriptive, each offering unique insights for informed decision-making.
Example 3: Predictive Analytics Report
Predictive analytics uses historical data to predict future outcomes. An example of a predictive analytics report might include:
- Sales forecasting for the next quarter based on past trends.
- Predictions of customer churn and strategies to retain valuable customers.
- Identifying potential future risks and opportunities in the market.
A predictive analytics report is often a game-changer for businesses, enabling them to make proactive decisions rather than reactive ones. Outbound Sales involves proactively reaching out to potential customers through calls, emails, and other methods to generate new business opportunities.
Example 4: Research Report Data Analysis
A research report data analysis involves examining data from scientific or market research to draw conclusions. For example:
- Analyzing survey data to understand customer satisfaction levels.
- Interpreting experimental data from a product development study.
- Providing insights into market trends based on research findings.
This type of report is often used by researchers, analysts, and businesses involved in market or product research. Lead Generation is the process of identifying and attracting potential customers through various strategies, helping drive sales and business growth.
Predictive Analytics Reporting
Predictive analytics has become a cornerstone of modern data-driven decision-making. By using predictive analytics tools, businesses can forecast future outcomes and make informed decisions based on data trends. The process involves data mining and statistical techniques to identify patterns in historical data and make predictions.
A predictive analytics data mining solutions report provides an in-depth look at the methods and technologies used to mine data for predictive insights. These reports might include:
- The types of data sources used (e.g., customer behavior, sales data, etc.).
- The algorithms applied to analyze and predict future outcomes.
- Recommendations on how businesses can leverage predictive analytics for specific business objectives.
A predictive analytics research report is typically produced by researchers or data scientists who have conducted a deep analysis of a business’s historical data to generate forecasts. The report might highlight trends in consumer behavior, market movements, or operational efficiency, providing the foundation for strategic planning. Omnichannel Customer Service provides seamless support across multiple channels, ensuring consistent and efficient customer experiences everywhere.
How to Create Data Analysis Reports
Creating an effective data analysis report requires a structured approach to ensure clarity and actionable insights. Here’s a step-by-step guide to writing a data analysis report:
- Define the Objective: Start by clearly identifying the goal of the report. Are you analyzing sales trends, customer behavior, or predicting future outcomes? Understanding the purpose of the analysis is crucial to focusing on the right data.
- Gather the Data: Collect the relevant data needed for your analysis. This could include sales records, customer interaction logs, or survey responses.
- Analyze the Data: Use appropriate data analysis tools and techniques to examine the data. This might involve statistical methods, machine learning algorithms, or simple descriptive statistics.
- Interpret the Results: Once the analysis is complete, interpret the findings. What patterns emerged? What trends are visible? What insights can you draw from the data?
- Generate the Report: Present the results in a structured format, including an introduction, methodology, findings, and recommendations. Be sure to include charts, graphs, and tables where applicable to illustrate key points.
- Make Recommendations: Based on your analysis, provide actionable recommendations for the business. These might include strategies for improving performance, addressing weaknesses, or capitalizing on opportunities.
At Rapid Phone Center, data analysis reports examples provide clear insights into business performance. These reports illustrate how raw data is transformed into actionable conclusions, offering businesses valuable examples of how to identify trends, optimize strategies, and enhance decision-making. Our comprehensive reports help companies drive growth and improve operational efficiency.
Best Practices for Data Analytics Reporting
An example of data analysis report from Rapid Phone Center demonstrates how raw data is carefully analyzed to uncover trends and insights. Our reports showcase how businesses can use data to improve decision-making, streamline operations, and optimize strategies. With clear visualizations and actionable conclusions, our reports empower companies to drive success and growth.
To ensure that your data analytics reports are effective, consider these best practices:
- Keep it Clear and Concise: Avoid jargon and overly technical language. Aim for clarity, and ensure that the report is easy for non-experts to understand.
- Focus on Actionable Insights: Provide insights that can lead to tangible actions. Decision-makers want to know how they can use the data to improve business outcomes.
- Use Visuals: Data visualization tools such as graphs, charts, and tables can help present complex data in an easily digestible format.
- Provide Context: Ensure that the data is contextualized. For instance, a sales data analysis report should include comparisons to past performance and industry benchmarks.
An example of a data analysis report at Rapid Phone Center showcases how detailed insights are derived from data, highlighting key trends and patterns. This report helps businesses make informed decisions by interpreting statistical data, offering valuable recommendations for optimizing strategies and improving overall performance.
Tools for Data Analytics and Reporting
At Rapid Phone Center, data analysis report examples highlight how data is transformed into actionable insights. These examples demonstrate the process of identifying key trends, making informed decisions, and optimizing strategies. Our reports help businesses leverage data to enhance performance, improve efficiency, and drive growth through clear, actionable conclusions and visualizations.
Several tools can help streamline the data analysis and reporting process:
- Tableau: A leading data visualization tool that allows users to create interactive reports and dashboards.
- Microsoft Power BI: A business analytics tool that helps visualize data and share insights across organizations.
- Google Analytics: Primarily used for web data analytics, Google Analytics provides reports on website traffic and user behavior.
- SAS: A powerful tool for statistical analysis and predictive modeling.
These tools can automate the process of data analysis and help generate detailed data analytics report.
Effective data analysis and reporting are essential for businesses to make informed decisions and stay ahead of the competition. Whether you’re generating a sales data analysis report, performing predictive analytics, or diving into big data analysis, the key is to present the data in a way that provides actionable insights. By leveraging the right tools and following best practices, you can ensure that your data analytics reports help drive business success.
Frequently Asked Questions
Explore Frequently Asked Questions for data analysis report examples at Rapid Phone Center to gain insights and improve your analysis process.
What is the purpose of this data analysis report?
The purpose of this report is to analyze and interpret data related to [specific topic, e.g., customer behavior, sales trends, market performance, etc.]. It aims to uncover meaningful patterns and insights to support decision-making and strategic planning.
How was the data collected for this analysis?
The data was collected through various methods, including surveys, transactions, sensor data, or third-party sources. Specific datasets used in this report include [briefly describe sources, e.g., company sales records, market surveys, etc.].
What analysis techniques were used?
The analysis employed various statistical techniques, such as regression analysis, hypothesis testing, clustering, and trend analysis. Data cleaning and preprocessing steps were performed to ensure accuracy and reliability.
How were outliers handled in the analysis?
Outliers were identified through statistical tests and visualizations such as box plots. In cases where outliers significantly impacted results, they were either removed or adjusted, depending on the context of the analysis.
You might also find our article intriguing:
Good Luck to You