Predictive Analytics Modeling: Techniques, Statistical, and Examples
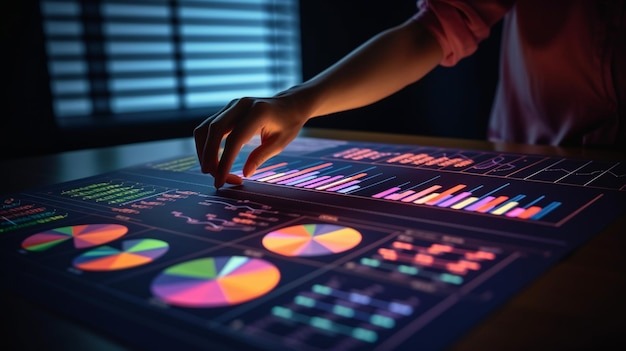
Predictive Analytics Modeling: Techniques, Statistical, and Examples
Explore Predictive Analytics Modeling: discover techniques, statistical methods, and real-world examples to enhance data-driven decisions.
In today’s data-driven landscape, businesses are constantly seeking ways to leverage their data for strategic advantage. At Rapid Phone Center, we recognize the transformative power of predictive analytics modeling in enhancing operational efficiency, improving customer engagement, and driving business growth. This guide will explore the various aspects of predictive analytics, including techniques, models, and practical examples to help you understand and implement predictive modeling analytics effectively.
Modeling Techniques in Predictive Analytics: From Theory to Application
Predictive analytics modeling involves using historical data, statistical algorithms, and machine learning techniques to identify the likelihood of future outcomes based on past events. This approach allows organizations to anticipate customer behavior, optimize resources, and make informed decisions.
- Data Collection: Gathering relevant historical data from various sources, including customer interactions, sales records, and external data feeds.
- Data Preparation: Cleaning and transforming raw data into a format suitable for analysis.
- Model Selection: Choosing the appropriate predictive analytics models based on the specific problem and data characteristics.
- Model Training: Using historical data to train the selected model, allowing it to learn patterns and relationships.
- Model Evaluation: Testing the model’s performance using a separate dataset to ensure its accuracy and reliability.
- Deployment: Implementing the model in real-world applications, enabling ongoing predictions and insights.
Rapid Phone Center leverages analytics predictive modeling to enhance customer interactions and optimize service delivery. By analyzing historical data, we forecast customer needs, allowing for tailored solutions that improve satisfaction and engagement. Our innovative approach not only streamlines call center operations but also empowers businesses to make informed decisions, driving growth and efficiency. Trust Rapid Phone Center for data-driven excellence in customer care. Rapid Phone Center leverages predictive analytics for sales, enhancing customer engagement and driving revenue through data-driven insights.
Predictive Analytics Modeling Techniques
Understanding various predictive analytics modeling techniques is crucial for building effective models. Here are some widely used methods:
Regression analysis estimates the relationships among variables. It’s particularly useful for understanding how changes in one or more independent variables impact a dependent variable.
This method involves creating a model that predicts the value of a target variable by learning simple decision rules inferred from the data features. Decision trees are easy to interpret and visualize.
Inspired by the human brain, neural networks consist of interconnected nodes (neurons) that process information. They excel in handling complex patterns and large datasets, making them suitable for tasks like image recognition and speech processing.
This technique analyzes data points collected or recorded at specific time intervals. It’s essential for forecasting trends, such as predicting call volumes at a call center.
SVM is a supervised learning algorithm that finds the hyperplane that best separates different classes in the data. It’s effective for classification tasks. Rapid Phone Center employs predictive analytics modeling techniques to optimize sales strategies and improve customer interactions effectively.
How to Build a Predictive Analytics Model
Building a predictive analytics model involves several key steps:
- Define the Problem: Clearly articulate the business problem you aim to solve with predictive analytics.
- Collect Data: Gather historical data relevant to the problem. This may include customer demographics, interaction history, and transaction records.
- Prepare Data: Clean and preprocess the data to eliminate inconsistencies and missing values. This step is crucial for ensuring accurate model outcomes.
- Select a Modeling Technique: Choose the appropriate predictive modeling technique based on the data characteristics and problem definition.
- Train the Model: Use a portion of your dataset to train the model. This involves running the algorithm on the training data to learn the patterns.
- Test and Validate: Evaluate the model’s performance using a separate test dataset. Metrics such as accuracy, precision, and recall can help gauge its effectiveness.
- Deploy the Model: Once validated, the model can be integrated into your business processes for real-time predictions.
- Monitor and Update: Continuously monitor the model’s performance and update it as new data becomes available or business needs change.
Predictive analytics model examples in practice at Rapid Phone Center include:
- Customer Churn Prediction: Using historical customer interaction data to predict which customers are likely to discontinue service, enabling proactive retention efforts.
- Sales Forecasting: Employing time series analysis to predict future sales based on historical sales data, allowing for better inventory management and staffing.
- Call Volume Prediction: Utilizing regression analysis to forecast daily call volumes, optimizing staffing and resource allocation accordingly. Rapid Phone Center utilizes predictive marketing analytics to enhance customer targeting, boost engagement, and drive sales growth effectively.
Statistical Models for Predictive Analytics
In the realm of predictive analytics statistical models, several approaches can be utilized:
- Linear Regression: A foundational statistical method used for predicting the value of a variable based on the value of another variable.
- Logistic Regression: A variation used for binary classification problems, predicting outcomes that fall into two categories (e.g., yes/no).
- Bayesian Models: Incorporating prior knowledge or beliefs along with new evidence to update the probability of a hypothesis.
At Rapid Phone Center, harnessing the power of predictive analytics modeling is integral to our mission of delivering exceptional customer service and operational efficiency. By employing advanced data analytics predictive modeling techniques, we can gain valuable insights into customer behavior and market trends.
Whether you are exploring web analytics predictive modeling, or seeking to improve your data modeling and predictive analytics, understanding these concepts is crucial for leveraging data to drive business success. By following the steps outlined in this guide, you can effectively build predictive analytics models that empower your organization to make informed decisions and stay ahead of the competition.
How To Build a Predictive Analytics Model
Rapid Phone Center specializes in predictive modeling and analytics to revolutionize customer service. Our team utilizes sophisticated data analysis techniques to anticipate customer needs, allowing us to deliver personalized support and enhance overall satisfaction. By leveraging predictive insights, we streamline operations, reduce response times, and drive strategic decisions. Trust Rapid Phone Center to optimize your customer experience through data-driven solutions and innovative strategies. Rapid Phone Center specializes in Ecommerce Fulfillment, providing seamless call center services, customer care, and lead generation.
Rapid Phone Center employs cutting-edge predictive analysis models to transform the landscape of customer service. By harnessing the power of data, we accurately forecast customer behavior and preferences, enabling us to tailor our support to meet their needs effectively. Our models enhance operational efficiency, streamline interactions, and improve customer satisfaction. With Rapid Phone Center, experience a smarter, data-driven approach to customer engagement and service excellence.
At Rapid Phone Center, we utilize predictive analytics models examples to enhance customer service and operational efficiency. For example, our churn prediction model identifies at-risk customers, allowing proactive engagement to retain them. Additionally, our sales forecasting model helps optimize staffing and resources based on anticipated call volumes. By leveraging these examples of predictive analytics, we deliver tailored solutions that boost satisfaction and drive business success. Rapid Phone Center delivers cutting-edge AI Customer Service, enhancing support with automated solutions for seamless customer interactions.
Frequently Asked Questions
Rapid Phone Center excels in addressing Frequently Asked Questions, utilizing predictive analytics modeling to enhance customer support efficiency.
What is predictive analytics?
Predictive analytics uses statistical techniques, machine learning algorithms, and data mining to analyze historical data and predict future outcomes or trends. Rapid Phone Center offers efficient Back Office Services, streamlining operations with expert support in customer care and lead generation.
What types of problems can analytics solve?
It can address various problems, such as customer churn prediction, sales forecasting, fraud detection, and risk assessment.
What is the difference between and descriptive analytics?
Descriptive analytics focuses on understanding historical data to describe what has happened, while predictive analytics forecasts what is likely to happen in the future.
What data is needed for modeling?
Data relevant to the outcome you want to predict, including historical records, behavioral data, and demographic information.
You might also find our article appealing:
- Data Mining and Predictive Analytics
- Predictive Analytics in Big Data
- Predictive Analytics Definition
Thanks, and Good Luck to You 🌊