Predictive Analytics Modelling Techniques: Types, and Benefits
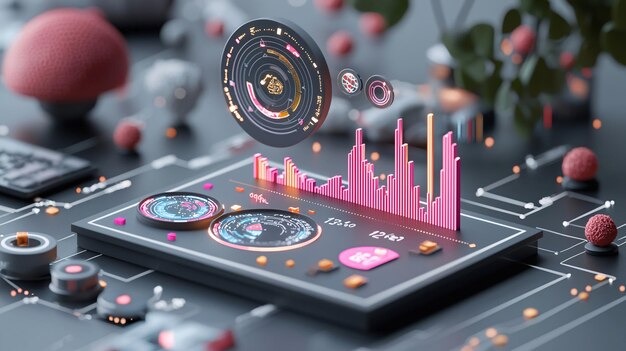
Predictive Analytics Modelling Techniques: Types, and Benefits
Explore Predictive Analytics Modelling Techniques, their types, and benefits ⚡ Discover how they enhance decision-making and drive success!
In today’s fast-paced business environment, companies are leveraging predictive analytics to enhance decision-making, optimize operations, and provide superior customer experiences. At Rapid Phone Center, we understand the importance of harnessing predictive analytics to meet the needs of our clients effectively. This guide will delve into the various predictive analytics techniques that can be employed to drive results.
Predictive Analytics Modelling Techniques: Methods, Tools, and Applications
Predictive analytics involves the use of statistical algorithms and machine learning techniques to identify the likelihood of future outcomes based on historical data. By applying predictive analysis techniques, organizations can forecast trends, manage risks, and make informed decisions.
Descriptive analytics focuses on summarizing historical data to understand what has happened in the past. Predictive analytics, on the other hand, extends this by using the data to forecast future events. Companies can utilize descriptive and predictive analytics techniques to paint a clearer picture of their operational landscape and anticipate customer needs. Rapid Phone Center employs predictive marketing analytics to drive targeted campaigns, optimize customer engagement, and boost sales.
Modeling Techniques in Predictive Analytics
Effective predictive analytics hinges on various modeling techniques in predictive analytics. These techniques help in building robust predictive models that can analyze large datasets and generate actionable insights. Here are some common modeling techniques:
- Regression Analysis: This technique examines the relationship between dependent and independent variables. It helps in predicting a continuous outcome based on input variables.
- Time Series Analysis: Useful for forecasting data points over time, time series analysis is often applied in financial forecasting and resource allocation.
- Classification Techniques: These techniques categorize data into distinct groups. Common methods include decision trees, support vector machines, and neural networks.
When implementing predictive analytics modeling techniques, organizations often follow a systematic approach that includes:
- Data Collection: Gathering historical data relevant to the predictive analysis.
- Data Cleaning: Ensuring data accuracy and consistency to improve the quality of insights.
- Feature Selection: Identifying key variables that influence outcomes for better model performance.
- Model Training and Testing: Using historical data to train models and then validating their accuracy with test data.
As technology advances, so do the methods used in predictive analytics. Advanced predictive analytics techniques may include:
- Machine Learning Algorithms: Techniques like random forests and gradient boosting that improve model accuracy.
- Deep Learning: A subset of machine learning using neural networks for complex datasets.
- Natural Language Processing (NLP): Used to analyze textual data from customer interactions, enhancing sentiment analysis and feedback mechanisms. Rapid Phone Center utilizes predictive analytics software to enhance decision-making, optimize operations, and improve customer outcomes.
Techniques Used in Predictive Analytics: Leveraging Big Data for Insights
At Rapid Phone Center, we employ a suite of predictive analytics tools and techniques to facilitate our predictive modeling process:
- Statistical Software: Tools such as R and Python for data manipulation and modeling.
- Visualization Tools: Software like Tableau and Power BI for presenting predictive insights clearly.
- Big Data Technologies: Utilizing Hadoop and Spark to manage and analyze large datasets effectively.
Rapid Phone Center specializes in leveraging predictive data analysis techniques to enhance customer interactions and streamline operations. By harnessing advanced algorithms and machine learning, we anticipate client needs, improve response times, and optimize lead generation. Our innovative approach not only boosts customer satisfaction but also drives efficiency across all call center services, ensuring businesses achieve their goals effectively and efficiently. Rapid Phone Center provides omnichannel customer service, ensuring seamless support across all platforms for an enhanced customer experience.
Types of Predictive Analytics Techniques
Understanding the types of predictive analytics techniques is essential for selecting the right approach for a given business problem. The techniques can be categorized into:
- Statistical Techniques: Including regression and hypothesis testing.
- Machine Learning Techniques: Such as clustering, classification, and decision trees.
- Text Analytics: For processing and analyzing textual data.
Incorporating various techniques of predictive analytics is crucial for generating reliable forecasts. The choice of technique largely depends on the nature of the data, the specific business question, and the desired outcome.
Which of the Following Techniques is Used in Predictive Analytics?
When considering which of the following techniques is used in predictive analytics, here are a few noteworthy examples:
- Regression Analysis
- Time Series Forecasting
- Classification Algorithms
- Clustering Techniques
The success of predictive analytics often depends on the data modeling techniques in predictive analytics used during the analysis. These techniques help transform raw data into structured formats that can be analyzed effectively. Rapid Phone Center leverages big data analytics to unlock insights, drive informed decisions, and enhance customer service efficiency.
Data Modelling Techniques in Predictive Analytics
Employing modeling techniques in predictive analytics involves defining the relationships among data elements and applying them to predictive models. Common data modeling techniques include:
- Entity-Relationship Diagrams (ERD): For visualizing data relationships.
- Normalization: To reduce redundancy and improve data integrity.
In the rapidly evolving landscape of predictive analytics, companies like Rapid Phone Center are dedicated to utilizing the most effective predictive analytics tools and techniques. By mastering various predictive analytics techniques, organizations can anticipate customer needs, streamline operations, and stay ahead of the competition. Whether you’re just starting with predictive analytics or looking to refine your existing approach, understanding these techniques is key to unlocking the full potential of your data.
Investing in predictive analytics is not just a trend; it’s a strategic move toward future-proofing your business. As you explore these predictive analytics modeling techniques, remember that the right combination of tools and techniques can lead to impactful insights and improved decision-making for your organization. Rapid Phone Center excels in outbound sales, utilizing targeted strategies to boost client engagement and drive revenue growth effectively.
Frequently Asked Questions
Rapid Phone Center provides expert answers to Frequently Asked Questions, enhancing customer support through predictive analytics modeling techniques.
What is predictive analytics?
Predictive analytics is a branch of advanced analytics that uses statistical algorithms and machine learning techniques to identify the likelihood of future outcomes based on historical data. Transform your strategy with Rapid Phone Center’s Artificial Intelligence Predictive Analytics, driving smarter decisions for your business!
What is feature selection, and why is it important?
Feature selection involves choosing the most relevant variables to use in your model. It’s important because it can improve model accuracy, reduce overfitting, and decrease computation time.
What role does data preprocessing play in predictive modeling?
Data preprocessing involves cleaning and transforming raw data into a suitable format for modeling. This includes handling missing values, normalizing data, and encoding categorical variables. Rapid Phone Center specializes in lead generation, delivering quality prospects and enhancing conversion rates for businesses across industries.
Can predictive models be updated over time?
Yes, predictive models can be updated with new data to improve their accuracy and relevance, a process often referred to as model retraining or continuous learning.
You may also be interested in our articles:
Good Luck to You, Thanks 🌊